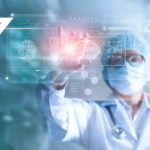
AI pioneer Innoplexus and phytopharmaceutical company DrD are partnering toRead More
AI pioneer Innoplexus and phytopharmaceutical company DrD are partnering toRead More
Apr 24
Mar 24
Innoplexus wins Horizon Interactive Gold Award for Curia App
Read MoreVisualize connections between biological entities using power maps and research graphs to discover potential drugs and targets.
Get access to real-time discovery, network analysis. and management of top and emerging key opinion leaders (KOLs) and digital opinion leaders (DOLs).
Optimize leadership strategy with real-time access to product performance, sales, budget and expenses, patient activity, CI landscape, etc.
Obtain up-to-date, relevant information and insights generated from continuously crawled multiple publicly available sources in one place.
Our AI and Blockchain technologies aim at empowering patients to create value with their own data. To achieve this goal, we created an ecosystem comprising all potential stakeholders (i.e. CROs, BioTech, Patients) in the entire drug discovery process that will/can benefit.
Via our Apps Curia for Cancer and Neuria for Neurologic diseases, Real World Data is being provided and utilized. (questionnaires) via Curia App & Neuria App Physicians Dashboards Hospital Dashboards
Cookie | Duration | Description |
---|---|---|
cookie-checkbox-analytics | 11 months | The cookie is used to store the user consent for the cookies in the category "Analytics". |
cookie-checkbox-functional | 11 months | The cookie is set to record the user consent for the cookies in the category "Functional". |
cookie-checkbox-necessary | 11 months | The cookies are used to store the user consent for the cookies in the category "Necessary". |
cookie-checkbox-others | 11 months | This cookie is used to store the user consent for the cookies in the category "Other. |
cookie-checkbox-performance | 11 months | The cookie is used to store the user consent for the cookies in the category "Performance". |
viewed_cookie_policy | 11 months | The cookie is used to store whether or not a user has consented to the use of cookies. It does not store any personal data. |
Computational framework to identify biomarkers using omics data constitutes a major part of the drug discovery, development and therapeutics. While diagnostic, prognostic, predictive, pharmacodynamic/ drug response, susceptibility/risk and safety biomarkers can be identified successfully using Innoplexus biomarker platform, RNAseq, HTS/ NGS, microarray, WES, proteomics and scRNAseq datasets can be used to identify genes, proteins or non-coding RNAs as potential biomarkers. Publicly available datasets from databases such as GEO Omnibus, ArrayExpress and PRIDE, etc, as well as client datasets can be integrated into biomarker pipelines. Beyond the analysis of surrogate endpoint or clinical endpoint biomarker analysis, Innoplexus platform provides deep-dive analysis into transcriptional regulation and cell specific differential expression.
Gene regulatory network analysis provides insights into transcription factors and their target interplay, also aids in understanding the hierarchy of transcription factors in a given network along with identification of master regulators. scRNAseq analysis platform enables investigations into distinct cell populations,their relative proportions and cell type specific differential expression of genes across different experimental conditions.
Discovery of molecules with desired properties such as i) selectivity for binding to a protein of interest, ii) novelty, iii) patentability and iv) satisfying drug-like properties such as solubility, bioavailability, non toxicity) is a risky and time-consuming task. Moreover, even with highest efforts, the success rate in identifying gene desirable molecules that succeed in a drug discovery pipeline is low.
At Innoplexus, we use an AI-driven approach to generate optimized molecular structures that facilitate drug discovery in the following ways:
As a consequence, our pipeline can quickly generate and shuffle through millions of molecules to identify the promising candidates and at the same time learn with each iteration to create better molecules with optimized drug-like properties thereby accelerating the drug discovery process.
Target identification is one of the most crucial steps in drug discovery because; almost half of the clinical trials fail due to inefficacy of selected drugs. One reason for this is inadequate target validation. Therefore, it is very important to understand and explore the role of all potential molecules involved in the disease pathophysiology.
Innoplexus leverages its AI-powered discovery engine to identify the most promising target with high biological relevance and market potential. Compelling advantages of AI-based processes are: An automation of manual and labor intensive tasks; An ability to process, integrate and make sense of large volumes of complex and unstructured datasets AI-enhanced process of knowledge discovery for understanding their cross-connections in biological networks. This is especially important in the early phases of drug discovery. AI can accelerate target identification processes and can also optimize identification and optimization of lead molecule discovery. AI does so by searching through the past knowledge of the compounds, by examining a large variety of combinations and by recommending the most suitable leads. In particular, deep learning methods can explore existing data to help predict how tissue or body systems may respond to a given drug.
Further, AI can play a role in the identification of stratified target patient populations for clinical trials, in predicting disease progression based on molecular data obtained from tissue samples and in stratifying patients either in the clinical trials preparation phase or in the optimization of the treatments based on patients’ responses and their individual characteristics.
Traditional methods of drug discovery and molecular optimization by iternative medicinal chemistry approaches is a time consuming, costly and laborious process. It takes ~12-15 years for a drug to reach the market and costs on average more than $1 billion USD. Identification of valid lead molecules is a key step in drug discovery and a tedious process along with a high failure rate. Of the many drugs that were discovered through traditional approaches, however only few of them reach the clinic. Most of them fail in the preclinical and clinical stage due to safety and efficacy issues. Hence, there is a huge need for AI-based models, which can predict bioactivity faster and in advance, so that money, effort and time can be saved by pharma and biotech companies in addition to improving treatment outcomes for the patients.
Prediction of a potential drug’s bioactivity and ADMET properties with high accuracy not only reduces the cost of the drug discovery process but also has a direct positive impact on an patient’s health. For example, if we estimate the bioactivity of a potential drug well in advance, we can predict whether it will be useful for further studies or not, thereby saving money, time and efforts.
Innoplexus has thus come up with a solution that leverages Cutting-Edge Technology to empower decision making. This involves development of an advanced SAR model consisting of transformer architecture ensembled with a deep learning (DL) based prediction model (state-of-the-art-method) to predict bioactivity of molecules. Also, PaDEL based descriptor models using traditional machine learning (ML) methods for bioactivity prediction have been developed, to enable the users to view and compare both ML and DL models, and thus select the best performing one based on the evaluation metrics. Further, SAR module also includes scaffold and lead generation sub-modules along with ADMET prediction and structure similarity sub-modules to ease the process of in silico drug design.
Identifying and prioritizing potential indications for selected targets is critical for clinical and commercial success. Given a target or drug, we leverage our proprietary algorithms and AI based technologies to identify most promising indications which are biologically significant and clinically feasible to conduct further clinical studies.
We use our artificial intelligence driven technology to discover, prioritize and market validate indications. We identify and filter across multiple dimensions, thousands of candidate indications by leveraging the Onto-Hypersearch capabilities of the Ontosight platform. We evaluate the importance of each indication, predict the likelihood of success and market-validate the best candidates based on competitive analysis.To discover and prioritize indication, our approach consists of the following steps:
At the end, our approach is able to prioritize the top go-to-market indications based on scientific facts, biological evidence, clinical feasibility and market potential.
Pharma companies spend more than 1 Billion USD on developing a new drug. Drug candidates pass the clinical stage with a success rate as low as 10-15%. Of all clinical studies, 86% fail to meet recruitment targets on time. Dropout rates of clinical trials commonly range between 15-40%. Of failed trials, 57% show limited efficacy i.e. due to poor statistical endpoints or underpowered samples and thus making clinical trials inherently more time consuming and expensive.
Predicting the probability or likelihood of success (PoS) for a clinical trial is important for pharma investors and to the clinical investigators that help them in evaluating the economic and scientific decisions. Without the proper estimate of PoS there is a chance for misjudging the risk and value proposition for the drug development that may lead to loss of opportunities for both investors and patients. Furthermore, to help prioritize the proper resource allocations, accurate and timely assessment of risk is critical and is the need of the hour for the drug development companies conducting the clinical trial. Innoplexus continuously crawls, aggregates, and structures data for the prediction engine by using the computer vision technology. The algorithms auto-label the clinical trials based on, if the clinical trial met, its endpoints to build a training dataset for the prediction engine. The predicted outcome of a clinical trial is based on its likelihood of meeting its endpoints. The model continually refines its predictions as more information is publicly disclosed.
Using the CTP engine, Innoplexus can help answer the following questions:
Discovering novel uses for existing drugs through drug repurposing can cut down the time and costs, and at the same time reduce the risk of failure associated with the development of new therapeutics. At Innoplexus we harness our AI/machine learning capabilities and corporate assets to identify and validate drug repurposing candidates at an unprecedented scale and depth in a modality-independent fashion. We integrate all relevant data from publicly available as well as proprietary resources to comprehensively assess approved drugs for their therapeutic utility and market potential in other indications. Repurposing existing drugs can decrease the cost and shorten drug development processes because many of the preclinical and clinical safety studies have already been completed before and after market approval. Drug repurposing can also improve the success rate of drug development because marketed drugs often have well-characterized safety and efficacy profiles across geographies and stratified patient cohorts
Such advantages are honored by regulatory authorities with shortened and expedited drug approval processes. At Innoplexus we tighten up the data-driven safety even further by validating drug repurposing candidates with AI-tailored predictive biomarkers to track the efficacy of repurposed therapeutics and with our AI-powered clinical trial prediction tool.